Unlocking the Future of Business with Innovative Data Annotation Tools
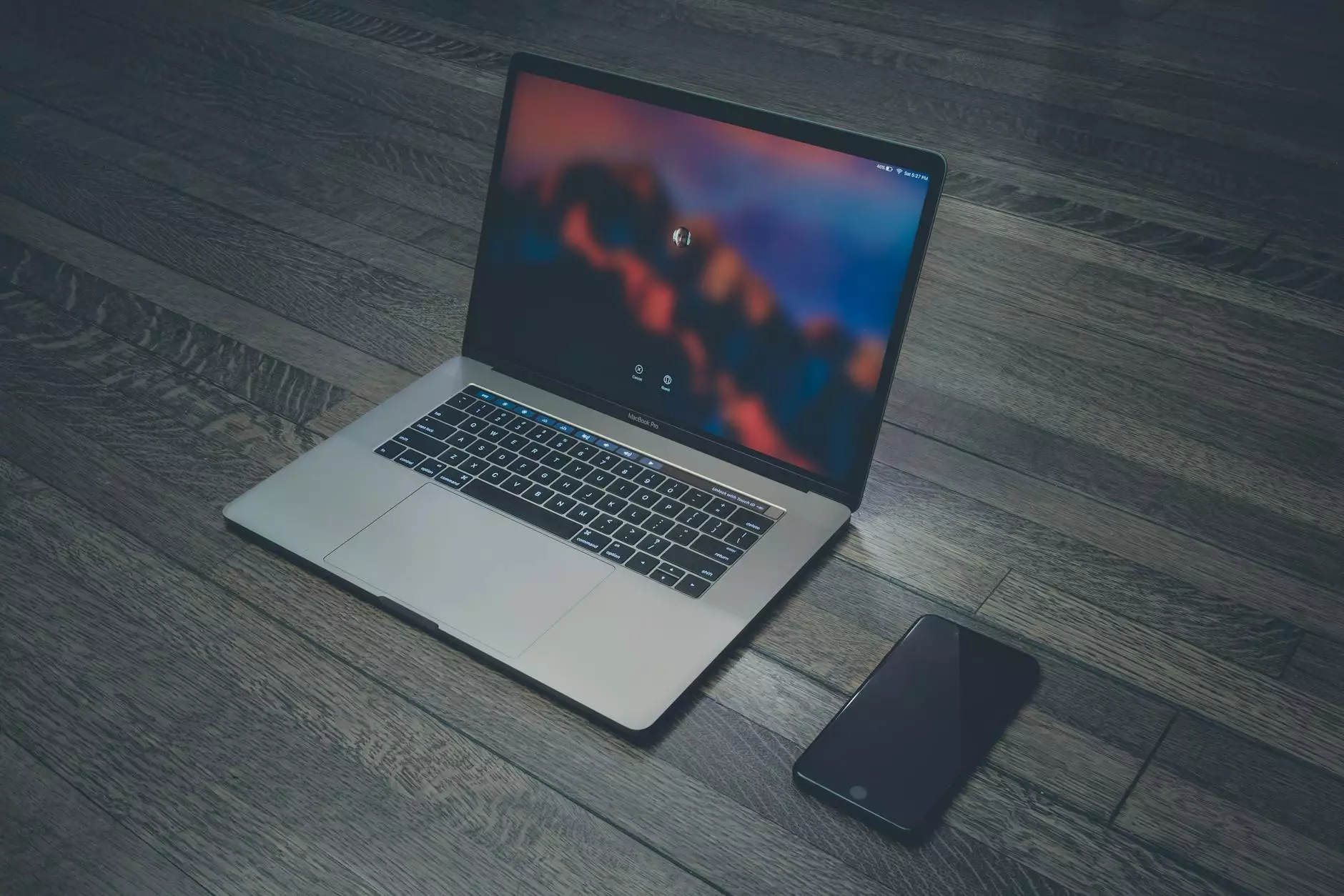
The modern business landscape is increasingly driven by data, and the ability to effectively manage and interpret that data can be the key to gaining a significant competitive advantage. At Keylabs.ai, we believe that our advanced data annotation tools and platforms can empower businesses to harness the full potential of their data. In this article, we will delve into the crucial role of data annotation in business, exploring its benefits, features, and applications to demonstrate how it can revolutionize operations and decision-making processes.
What is Data Annotation?
Data annotation is the process of labeling or tagging data to make it understandable for machine learning algorithms. This technique is pivotal in training AI models, which rely on high-quality annotated data to learn patterns and make predictions. The significance of data annotation in today’s tech-centric world cannot be overstated.
The Importance of Data Annotation in Business
Incorporating a robust data annotation strategy can lead to numerous advantages for businesses across various industries. Here are some essential benefits:
- Enhanced Machine Learning Models: Well-annotated data feeds machine learning algorithms, enabling them to learn and improve their accuracy over time.
- Improved Decision Making: Data annotation allows businesses to derive insights from data, leading to informed strategic decisions.
- Increased Efficiency: Automated data annotation tools can drastically reduce the time and effort involved in data preparation.
- Quality Control: Annotation processes can ensure the quality and consistency of data, which is crucial for achieving reliable outcomes with AI systems.
Types of Data Annotation Tools
Data annotation is not a one-size-fits-all approach. Different tasks require tailored solutions. Here are some key types of data annotation tools available in the market:
- Image Annotation Tools: Used for object detection, image segmentation, and classification in computer vision applications.
- Text Annotation Tools: Essential for natural language processing (NLP) tasks, including sentiment analysis and entity recognition.
- Audio Annotation Tools: Useful for transcribing audio data and identifying speakers in audio analysis applications.
- Video Annotation Tools: Vital for developing models that process video content, including action recognition and content tagging.
Key Features of a Comprehensive Data Annotation Platform
A forward-thinking data annotation platform, such as Keylabs.ai, typically offers a range of features designed to expedite the annotation process while ensuring high quality and reliability. Here are some features to consider:
- Intuitive Interface: User-friendly design facilitates seamless use, even for individuals without technical expertise.
- Collaboration Tools: Allow teams to collaborate in real-time, sharing insights and improving annotation efficiency.
- Multiple Annotations: Support for various types of annotations, including bounding boxes, polygons, and points to cater to diverse needs.
- Integration Capabilities: Ability to integrate with existing machine learning frameworks and data management tools, ensuring a smooth workflow.
- Quality Assurance: Built-in quality control features, such as review processes and validation checks, to maintain high annotation standards.
The Role of Machine Learning in Data Annotation
As businesses adopt machine learning to enhance their operations, the reliance on data annotation grows. Machine learning models can learn from annotated data, allowing them to improve over time and make predictions based on new, unannotated data. With the integration of AI in data annotation, companies can expect:
- Automated Annotation: Reducing human effort by using pre-trained models to perform annotations faster and at scale.
- Active Learning: Streamlining the annotation process by prioritizing the most informative data for human annotators to label.
- Continuous Improvement: Machine learning models can continuously refine their predictions as they access more annotated data.
Implementing a Data Annotation Strategy
To successfully implement a data annotation strategy within your organization, consider the following steps:
1. Define Your Objectives
Identify the specific use cases for data annotation within your business. Are you looking to enhance a machine learning model for customer segmentation, or do you need to improve your NLP capabilities for customer service automation?
2. Choose the Right Tools
Select a data annotation tool or platform that suits your specific needs. Explore the offerings of Keylabs.ai to find features that align with your objectives.
3. Train Your Team
Ensure that your team is well-trained in using the chosen annotation tools. Conduct workshops or training sessions to enhance their capabilities and understanding of the annotation process.
4. Implement Quality Control Measures
Quality control is critical. Implement review processes to ensure the annotated data meets the required standards before it is used for model training.
5. Evaluate and Iterate
Continuously assess the effectiveness of your annotation strategy. Use performance metrics to evaluate the impact of annotated data on your machine learning models.
Real-World Applications of Data Annotation
Data annotation is versatile and can be applied across various sectors. Here are some real-world applications:
Healthcare
In healthcare, annotated data is crucial for training models to diagnose diseases, predict patient outcomes, and personalize medicine. For instance, annotated medical imaging data can help radiologists identify anomalies with greater accuracy.
Autonomous Vehicles
Data annotation plays a pivotal role in the development of self-driving cars. Annotated images and video feeds help vehicles recognize objects, navigate roadways, and respond to dynamic environments safely.
Retail and E-commerce
In the retail sector, data annotation helps in analyzing customer behavior, managing inventory through demand forecasting models, and improving customer service with automated chatbots powered by natural language understanding.
Finance
Financial institutions leverage data annotation for fraud detection systems, risk assessment models, and personalized customer experiences through advanced recommendation systems.
Challenges in Data Annotation
While data annotation is advantageous, it is not without challenges. Understanding these obstacles can help businesses devise strategies to overcome them:
- Scalability: As data volumes grow, maintaining consistent quality across vast datasets becomes challenging.
- Labeling Accuracy: Ensuring accurate annotations requires skilled annotators, which may limit throughput and increase costs.
- Data Privacy: Protecting sensitive information during the annotation process is crucial, especially in regulated industries like healthcare and finance.
The Future of Data Annotation
As we look ahead, the data annotation landscape is poised for remarkable transformations driven by technology:
- Artificial Intelligence: AI-driven annotation tools will become more prevalent, allowing for faster and more accurate data labeling.
- Crowdsourcing: Leveraging crowdsourced annotation can enhance scalability while introducing diverse perspectives into the annotation process.
- Enhanced Collaboration: Future platforms will foster greater collaboration among cross-functional teams to streamline data gathering and annotation tasks.
Conclusion
In summary, effective data annotation is a cornerstone of successful business operations in the age of AI and big data. By utilizing advanced data annotation tools and platforms like Keylabs.ai, organizations can unlock valuable insights, enhance the quality of their machine learning models, and drive innovation across various sectors. Whether in healthcare, finance, or retail, the applications and benefits of data annotation are boundless. Embrace this transformative process to position your business for success in a data-driven future.
https://keylabs.ai/