Unlocking Business Potential with Machine Learning Annotation
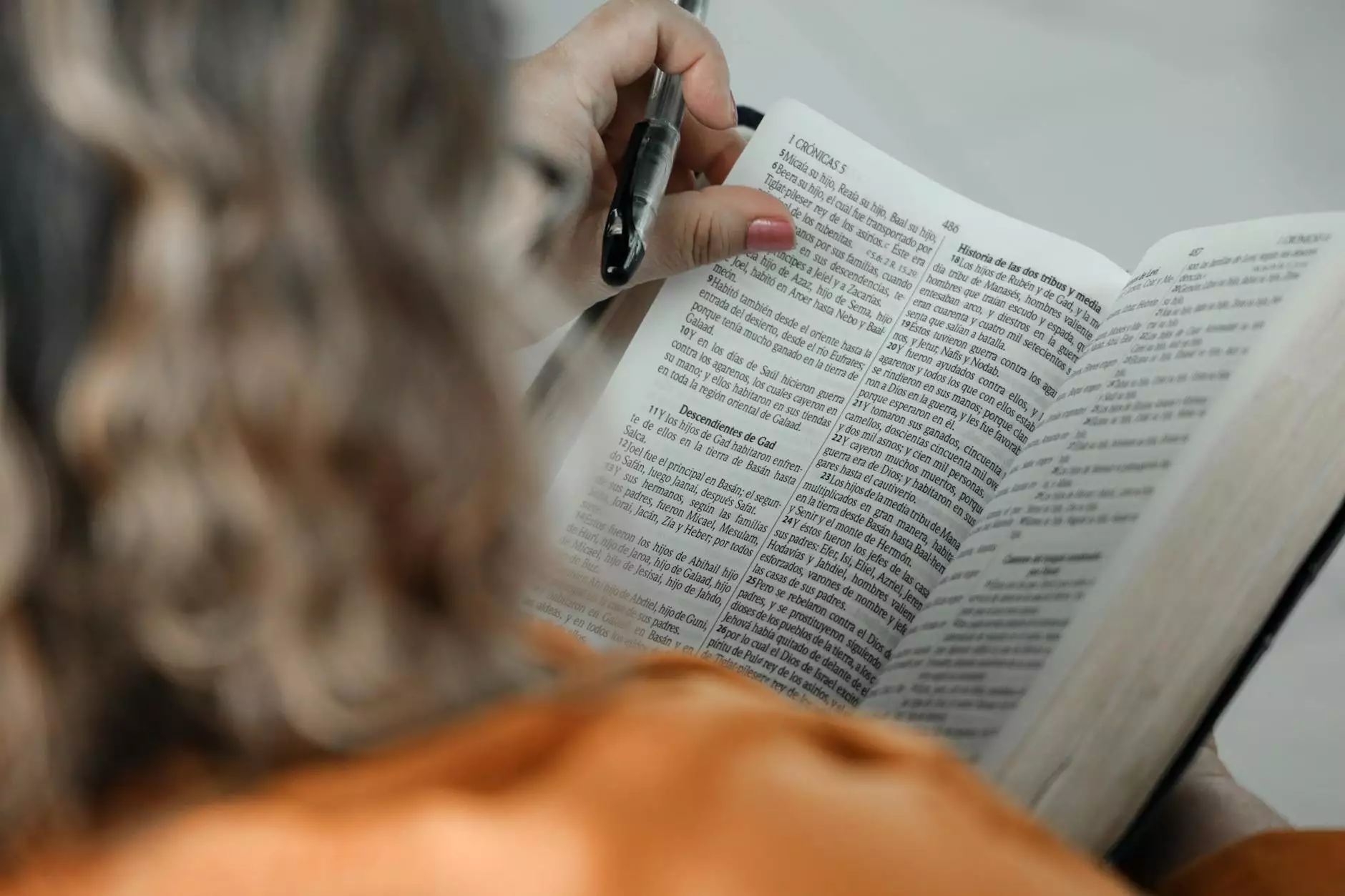
In today's fast-paced business landscape, the application of advanced technologies is crucial to maintaining a competitive edge. One of the most transformative technologies is machine learning annotation, which plays a vital role in optimizing operations across various industries, including Home Services, Keys & Locksmiths. This article explores the significance of machine learning annotation, its business applications, and how it can elevate your business performance.
What is Machine Learning Annotation?
Machine learning annotation refers to the process of labeling data to train machine learning models. This involves identifying specific features in datasets and marking them for the model to recognize patterns and make informed predictions. By providing structured, annotated data, businesses can enhance the performance of their machine learning applications.
The Importance of Machine Learning in the Business Sphere
As businesses increasingly rely on data-driven decisions, the integration of machine learning has become indispensable. Various sectors have adopted machine learning for its ability to:
- Increase efficiency: Automating tasks and processes can significantly reduce time and costs.
- Enhance customer experience: Personalized services lead to higher customer satisfaction and loyalty.
- Provide actionable insights: Data analysis offers deeper understanding and foresight into market trends.
- Predict outcomes: Anticipating customer behaviors and market fluctuations allows for proactive strategies.
Why Annotation is Crucial for Machine Learning Models
The success of machine learning heavily relies on the quality of the data fed into models. Here's why machine learning annotation is vital:
- Data Quality: High-quality, accurately annotated data enables models to learn effectively.
- Model Precision: Well-labeled data enhances the precision of predictions, reducing the margin of error.
- Scalability: Growing businesses can scale their operations by leveraging annotated datasets for continuous improvement.
- Versatility: Annotated data can be used across different models and applications, providing flexibility.
Applications of Machine Learning Annotation in Home Services
Home services businesses can leverage machine learning annotation in various ways to optimize their operations:
1. Predictive Maintenance
By annotating data collected from sensors and devices, businesses can predict when maintenance is due, minimizing downtime and reducing repair costs.
2. Customer Interaction and Support
Through machine learning models trained on annotated customer interactions, businesses can improve their AI-driven chatbots and customer service applications, ensuring prompt and accurate responses.
3. Marketing and Customer Acquisition
Data annotation allows businesses to analyze customer behavior patterns, leading to more targeted marketing strategies and effective customer acquisition campaigns.
Impact of Machine Learning Annotation on Locksmiths
The keys and locksmiths industry can also significantly benefit from machine learning annotation:
1. Secure Access Control
Annotated data from security systems can enhance access control mechanisms, providing more robust security solutions for clients.
2. Inventory Management
By utilizing machine learning for inventory management, locksmiths can forecast demand more accurately and optimize stock levels, leading to cost savings and improved service delivery.
3. Fraud Detection
Annotated datasets can be employed to train models that detect fraudulent activities, protecting both businesses and customers from potential threats.
Steps to Implement Machine Learning Annotation in Your Business
Implementing machine learning annotation can seem daunting, but with a systematic approach, businesses can seamlessly integrate this powerful tool:
1. Identify Your Objectives
Start by defining what you hope to achieve with machine learning annotation. This could range from improving customer service to enhancing security measures.
2. Collect Data
Gather the relevant data required for your machine learning model. Ensure that this data is comprehensive and representative of the scenarios you wish to automate or enhance.
3. Choose the Right Annotation Tools
There are numerous tools available for data annotation. Select one that fits your business needs, considering factors like scalability, ease of use, and integration capabilities.
4. Annotate the Data
If possible, involve subject matter experts in the annotation process to ensure accuracy. Alternatively, consider outsourcing to professional annotation services if in-house resources are limited.
5. Train Your Machine Learning Model
Once your data is annotated, you can begin training your machine learning model. Monitor its performance and adjust your strategies as necessary.
6. Evaluate and Iterate
After deployment, continue to evaluate the model's effectiveness. Gather feedback and make iterative changes to enhance performance.
Challenges in Machine Learning Annotation
While the benefits of machine learning annotation are significant, businesses may encounter challenges such as:
- Data Privacy: Ensuring compliance with data protection regulations can be an obstacle in data collection and annotation.
- Resource Allocation: Data annotation can be time-consuming and may require additional personnel or tools.
- Consistency: Maintaining consistency in data labeling is crucial and can be challenging without proper guidelines.
Future Trends in Machine Learning Annotation
The landscape of machine learning annotation is continuously evolving. Here are some trends to watch:
- Automated Annotation: The rise of AI-powered annotation tools, which can automate portions of the annotation process, will streamline workflows.
- Real-time Data Annotation: Businesses will increasingly adopt real-time annotation capabilities, allowing instantaneous data labeling for enhanced agility.
- More Collaborative Annotation Platforms: Expect to see more platforms that enable teams to collaborate efficiently on data annotation projects.
Conclusion
Machine learning annotation is a crucial component of successful data-driven strategies in the Home Services and Keys & Locksmiths industries. By understanding its significance and implementing effective annotation methods, businesses can unlock greater operational efficiency, enhance customer experiences, and drive growth. Investing in machine learning annotation offers a pathway to future-proof your business and stay ahead in an increasingly competitive market.
As you consider integrating machine learning into your business strategy, remember that the quality of your annotated data is paramount. By prioritizing high-quality annotations, you pave the way for success in your machine learning initiatives.